Human beings are known to be especially capable of doing things that other species might not be able to accomplish during our lifetime. Oftentimes, we solve questions that are seemingly impossible to answer and yet time and time again we find ourselves beyond what our ancestors might not be able to do.
Doing so may require the joint effort of hundreds, thousands, or even millions of people. You see, usually, these kinds of questions or problems that involve peering internally into human beings are typically the harder problems to solve. Consciousness, intelligence, understanding the mechanics of human life — these things that are internally within us seem to be the most complex of questions out there.
A problem that lies similarly in the spectrum of difficult questions is the problem of protein folding. As a fundamental physical process that defines human life and life in general, understanding how proteins fold is certainly of great importance for reasons we are going to discuss later. However, it remains a mystery as to how their mechanics truly work despite being so essential to living.
This article will discuss the ongoing efforts by scientists and researchers alike to understand and solve protein folding. But before anything, I will introduce some core concepts to better understanding the importance of protein folding, what it is, and how we can contribute to a global effort of improving human life using our personal gaming rigs.
Proteins and Protein Folding
Let’s start with asking the question of why understanding proteins are important. They, almost literally, are the building blocks of life. Our bodies are made up of different organs and tissues, which are made up of cells, which are made up of organelles. These organelles are made of four major groups: carbohydrates, lipids, nucleotides, and proteins — being the most complex biological compound out of the four.
Now, what do proteins actually do? Different proteins have their own purposes and functions, but it’s important to know that their shape is a major factor that decides the function. The shape of the active site of enzymes, which are made of proteins, for instance, determines which molecules would be able to bind.
In a similar fashion, an antibody, a protective protein produced by the immune system in response to the presence of a foreign substance (antigen), has a binding site whose shape fits a specific epitope. Simply put, the shape determines whether the weapon of choice would be effective in battling specific enemies.
It is hence quite clear that proteins are a necessity to human life, but that doesn’t necessarily translate to us comprehending how they behave, including how they fold themselves. A protein’s activity is greatly determined by its three-dimensional (3D) structure, which is produced as a result of folding.
So what is protein folding? Originally, a protein is just a sequence of amino acids before they fold. But in order to biologically function, they are thus folded or converted into 3D structures. Different proteins have different 3D structures, as they require different structures to stabilize and function.
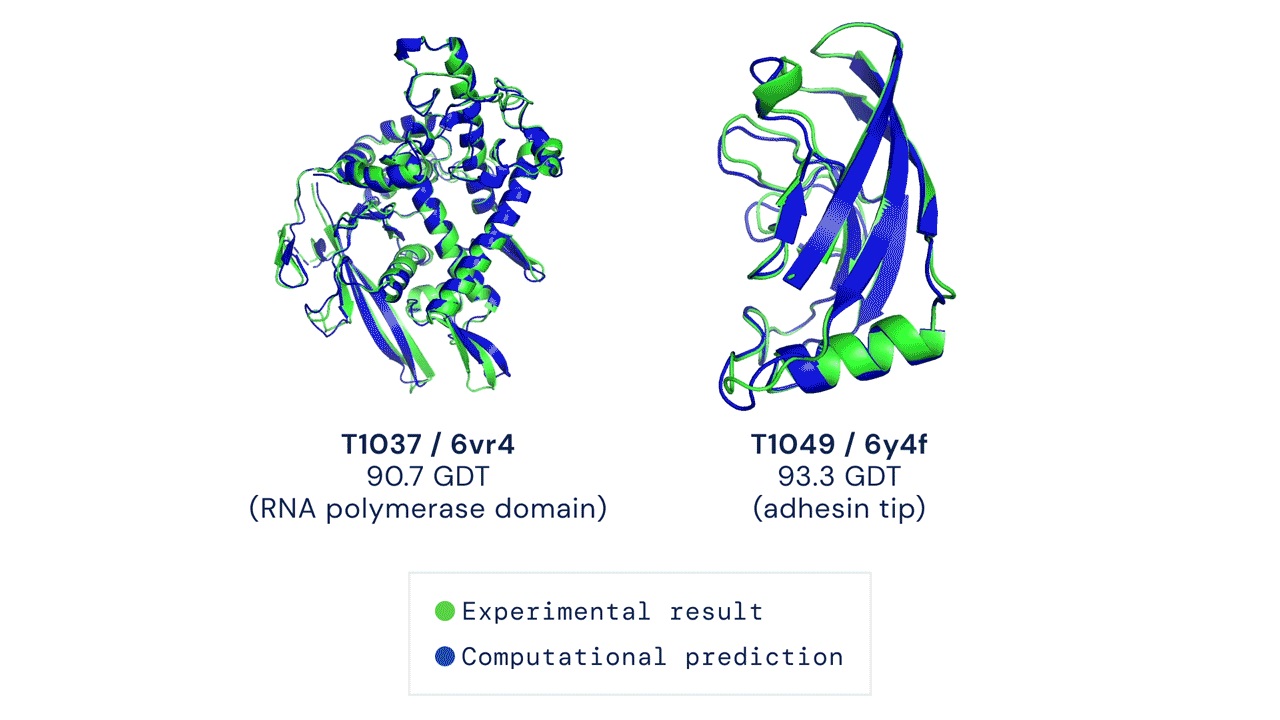
It is this very problem of how these end 3D structures are formed that remains as the big question mark. At this moment, there are over 200 million known proteins across all life forms, but only 170,000 protein structures are known to us over the last 60 years of studying them (Service, 2020).
Explaining how the underlying folding process actually works is a bit too ambitious at the moment. What scientists and researchers are currently trying to do is to at least, somehow, predict a protein’s end 3D structure given its sequence, instead of interpreting how they were formed.
By at least knowing the resultant structure, we would know what the protein’s functionality is like and analyze details about it. Through techniques like X-ray crystallography, cryo-electron microscopy, and nuclear magnetic resonance, we can determine the structure of proteins. And so scientists do these experiments in the lab, which are painstakingly slow and costly processes.
Therefore, there are efforts to automate this process more efficiently while maintaining accuracy, through different methods of computation. Traditionally, the molecular dynamics of protein folding are simulated in computers. The simulation keeps going until a stable structure is achieved which might reflect how the proteins truly are naturally.
However, the main drawback to this method is how large the search space of possibilities is. It takes only a millisecond for a protein to fold, but randomly simulating all the possible resultant structures to reach the ground truth would take longer than the age of the known universe (DeepMind, 2020). There’s virtually no exaggeration to this statement, as it is discussed in what’s known as Levinthal’s paradox.
The Problem with Protein Folding
As mentioned earlier, proteins are made up of chains of amino acids. Short chains of amino acids are known as peptide chains. These peptide chains are linked to one another by peptide bonds and these bonds could be configured differently to produce different resultant structures of proteins.
More specifically, these peptides bonds could be configured to have different angles which translate to the majority of the large search space. Levinthal estimates that there is an astronomical number of possible conformations of an unfolded peptide chain.
In his paper (Levinthal, 1969), he wrote, “How accurately must we know the bond angles to be able to estimate these energies? Even if we knew these angles to better than a tenth of a radian, there would be 10300 possible configurations in our theoretical protein.”
Moreover, it’s important that to understand why the correct folding of proteins is crucial. An incorrect fold of a protein is known as misfolding. Surprisingly, the effects of the misfolding of proteins could instead backfire on its host, known as proteopathy.
Diseases such as Huntington’s disease, Alzheimer’s disease, and Parkinson’s disease, have been shown to closely correlate to the event of protein misfolding (Walker & Levine, 2020). Further, others also suggest that cellular infections through viruses like influenza and HIV involve folding activities on cell membranes (Mahy et al., 1998).
Therefore, if we can better understand how misfolding works, we can help mitigate the effects of protein misfolding through therapies that could either destroy these misfolded proteins or assist the right folding process (Cohen & Kelly, 2003). All in all, understanding how proteins fold will benefit us for the better by not only providing novel insights, but also tackle ongoing health problems we face.
Large-Scale Computational Projects
Before the rise of machine learning methods to tackle the problem of protein folding, most approaches rely on molecular dynamics simulation to find the resultant 3D structure of proteins. However, as outlined by Levinthal, a more clever strategy is required, otherwise, the problem is very much unsolvable within finite time.
One particular joint effort that became massively popular due to the COVID-19 pandemic is what’s known as large-scale computational projects. These projects allow volunteers across the world to assist the modeling of protein folding through distributed computing. That is, volunteers would lend their local computing power to these projects via the internet.
So almost literally, a ton of people are accumulating their personal computing resources to build one giant supercomputer, to be used to model the very expensive and lengthy protein folding simulation. A few of the ongoing projects which implement the channel of distributed computing include Folding@home, Rosetta@home, and Foldit.
What’s interesting is that software like Folding@home requires the same computational power used to render 3D video games, specifically those requiring a heavy utilization of Graphical Processing Units (GPU). CPUs would also do the job, but only multi-core processors are the one that really makes the cut. Who would have thought that gaming powerhouses were as useful for advancing science?
In fact, Folding@home used to work on PlayStation 3s through an online application called Life with PlayStation. More than 15 million gamers participated in the program, accumulating a whopping 100 million computation hours over the course of five years and seven months. Sadly, this game-changing approach was concluded in 2012 with the Life with PlayStation to cease service.
Of course, even if we have the largest supercomputer on Earth, random calculations of possible protein folds are implausible, again by Levinthal’s idea. Folding@home, particularly, approached this task a little more strategically than just outright wasting the supercomputing resource that they have gathered from thousands, or perhaps millions of computing power.
According to the Folding@home team, their technique’s core idea is the modeling of protein folding using Markov State Models (MSM). Essentially, what Markov State Models do is to explore all the possible configurations more efficiently by avoiding redundancy.
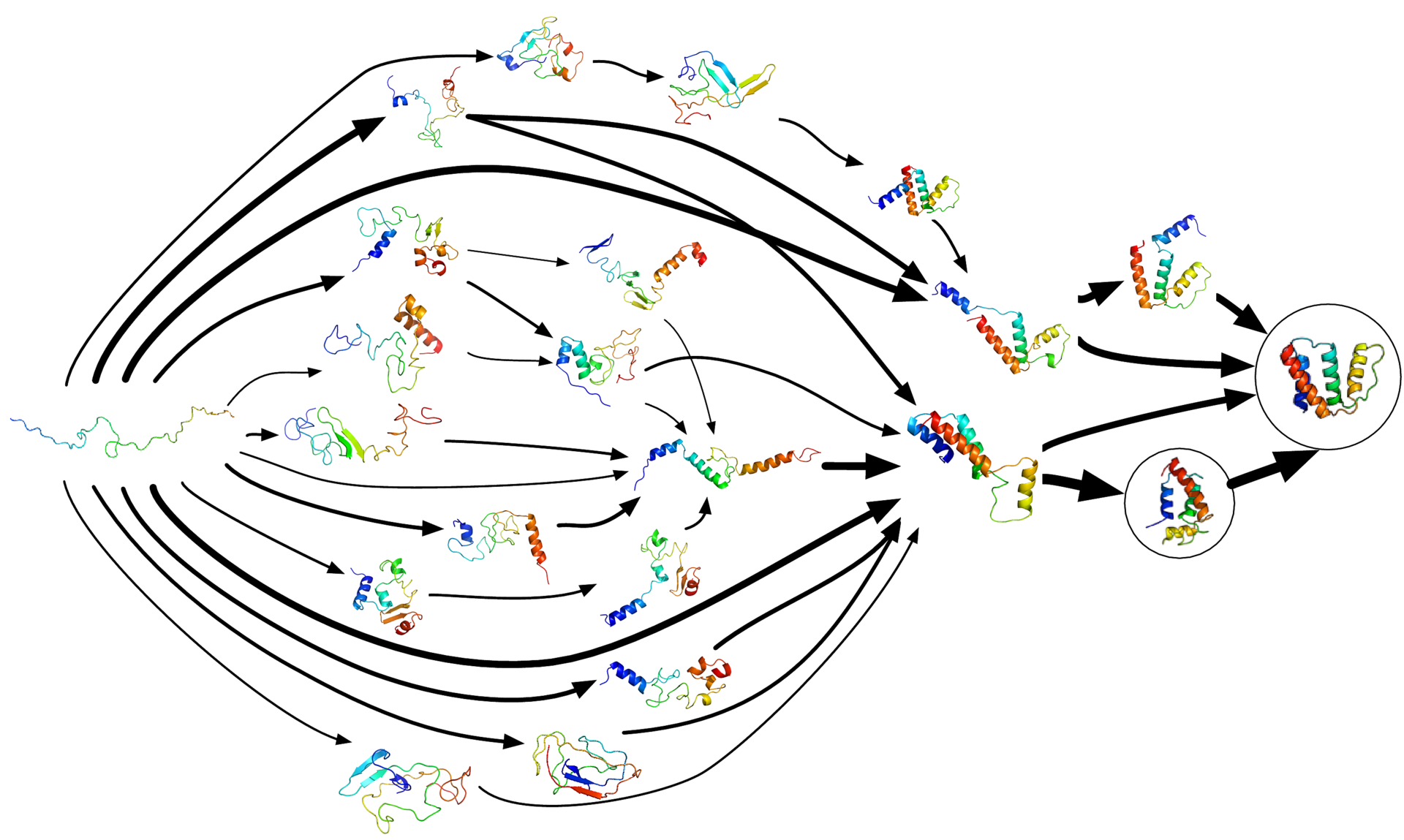
As highlighted on their FAQ page, Markov State Models are “a way of describing all the conformations (shapes) a protein – or other biomolecule for that matter – explores as a set of states (i.e. distinct structures) and the transition rates between them.”
Aside from only exploring distinct structures, Markov State Models are much better suited for parallel/distributed computing, like that of the Folding@home project. They allow for “the statistical aggregation of short, independent simulation trajectories” which “replaces the need for single long trajectories, and thus has been widely employed by distributed computing networks”.
Further, Folding@home employs a modified approach to the classic Markov State Model, by applying the technique of adaptive sampling. Technically speaking, adaptive sampling builds the model on the fly as the data is being generated, rather than building the model only after the data has been collected.
The FAQ page elaborates on the advantages of adaptive sampling in a simple analogy of maze exploration using the aid of GPS. To put it differently, imagine you’re roaming around an open RPG world. If the world is massive and you’re tasked to find the best resources, revisiting the same site over and over again would be very inefficient, wouldn’t it?
With the GPS, you can keep watch of where you’ve been while building a map, avoid revisiting the same places, and effectively gather the best resources maximally; that’s adaptive sampling in a rough sense. There’s more explanation behind the idea of adaptive sampling in Markov State Models, which I strongly suggest the readers dive into the technical details if the topic greatly interests you.
Folding@home is one of the largest worldwide efforts to help tackle the problem of protein folding. It even has some of the leading figures in the world as its ambassadors, in a time when collaboration is as important as ever. The list includes Microsoft CEO Satya Nadella, AMD President & CEO Dr. Lisa Su, NVIDIA CEO Jensen Huang, Intel CEO Bob Swan, and still many other relevant people.
Of course, other similar projects like Rosetta@home and Foldit have their own approaches to protein folding. Rosetta@home, for instance, doesn’t address the question of how and why proteins fold like Folding@home attempts to answer. Instead, it concentrates on computing protein design and predicting protein structure and docking.
Nevertheless, these efforts complement each other and could utilize each other’s strengths to advance their own endeavors. For example, Rosetta@home’s conformational states could become the starting point for Folding@home’s Markov State Model. Likewise, Rosetta@home’s protein structure prediction could be verified by Folding@home’s simulation.
It’s safe to say that although both projects handle the problem differently and try to answer different sets of questions, they ultimately benefit the study of protein folding.
Machine Learning Methods
In recent years and with the advancement of machine learning methods, the problem of protein folding has found yet another probable solution. Instead of simulating the folding of proteins like the attempts explained earlier, some machine learning methods are used to predict the end structure of the protein given its sequence.
One of the more famous attempts that made it to mainstream headline is AlphaFold 2, a program built by Google’s DeepMind. The AlphaFold team joined what’s known as the CASP (Critical Assessment of protein Structure Prediction) competition which serves as a blind assessment of how well a program/model is able to predict the 3D structure of proteins (Moult et al., 1995).
In 2018, DeepMind began to join the CASP competition, starting with CASP13. The company is already famous for its achievements with AlphaGo, so it was definitely intriguing to see whether they could emulate the same successes in a completely different realm of protein folding. Of course, being an AI company, DeepMind naturally entered the competition using their machine learning/AI methods.
Unsurprisingly, the AlphaFold team managed to outperform every other team in the CASP13 competition using their unconventional methods. According to their paper (Senior et al., 2020), the team used very deep residual networks (He et al., 2016) — the same method used widely for image recognition and various deep learning tasks — coupled with evolutionary profiles (Pellegrini et al., 1999). AlphaFold 1 achieved a median global distance test (GDT) score of 58.9 out of 100.
Then in 2020, a large part of the active community was shocked to see how well AlphaFold 2 performed in the proceeding CASP14. AlphaFold 2 didn’t only win CASP14, they too attained a median GDT of 92.4 which is as good as the gold standard results found by using experimental methods like X-ray crystallography and cryo-electron microscopy.
Although the official AlphaFold 2 paper, and hence method, hasn’t been fully released, the team revealed the general outline of their approach. Their approach includes the usage of the attention mechanism (Bahdanau, Cho, & Bengio, 2014), a technique surprisingly commonly used for natural (human) language (Vaswani et al., 2017). In essence, this mechanism calculates the relation between two components in a sequence.
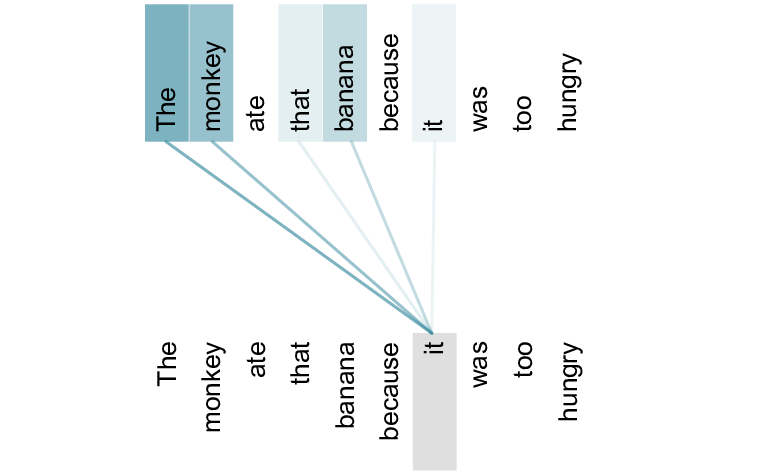
In natural language, this would translate to finding relationships between words in a sentence. For example, given the sentence “the monkey ate that banana because it was too hungry”, the attention mechanism would highly correlate the words ‘monkey’ and ‘it’, while the words ‘banana’ and ‘it’ would have a lower correlation.
Likewise, the same attention mechanism has the potential to capture the relationship between an amino acid residue of the protein and another amino acid residue. In a different paper by Vig et al. (2020), the authors have shown that the attention mechanism can indeed draw the relationship between the folding structure of proteins, targets binding sites, and “focuses on progressively more complex biophysical properties with increasing layer depth”.
Therefore, what DeepMind managed to do using these existing tools in the seemingly unrelated fields of language and protein folding showed the possibility of finding parallels between the nature of data. Unfortunately, there are some drawbacks to using machine learning models, and molecular simulations in general.
For one, these machine learning methods are tough to interpret and it is the same ongoing problem with other tasks that rely on machine learning models. These algorithms almost serve as a black box that we have yet to be able to peer into, a problem known as Explainable AI (XAI). Secondly, AlphaFold was only tested on small protein domains, while a large part of proteins consists of multi-protein complexes.
Finally, although the AlphaFold 2 was able to achieve a GDT score of over 90%, the error rate that the program exhibits is still currently unusable in laboratories for usages like drug design, etc. Nevertheless, the last two problems seem the easiest to solve for the next rendition of AlphaFold. A major factor to these issues is mostly derived from the fact that the existing data for protein folding is scarce and machine learning methods could better generalize given the increase in the availability of data.
One thing to note is that, like molecular simulation, AlphaFold took a ton load of computational resources to train. DeepMind reported that the computing power used equated to that of 100 to 200 GPUs, spanning a total of “a few weeks” to finish.
In general, however, whichever path you decide to take, be it molecular simulations or machine learning methods, these two approaches can only partially emulate what’s truly happening in reality. In other words, even if we could solve the protein folding problem via these computations, they might not necessarily reflect natural proteins in the real world. Thus, more checking and surely clinical trials of these methods are further required.
Doing Our Part
For the reasons discussed above, it should be quite clear that protein folding is a serious matter and would require the efforts of many to devise a solution that would answer one of humanity’s biggest questions ever. Healthcare, drug design, and virus protein structure analysis are only some of the byproducts of being able to solve protein folding.
The ongoing pandemic is living proof that what we can do together would greatly improve the wellbeing of everybody around us, even in the smallest ways. If you feel strongly about this topic, I strongly encourage you to learn more and read various relevant resources on the matter to better understand, empathize, and educate ourselves and the people around us.
Since its launch in October 2000, results from Folding@home have helped scientists at Pande Lab produce 225 scientific research papers, all of which accord with experiment results. And if you have a gaming rig with insane GPU setups lying around doing nothing, I’d suggest you put it to good use, for a purpose that could improve the lives of many, including yours. Try a few of the large-scale computation projects like Rosetta@home, Folding@home, or whichever’s your favorite; let’s do our part.
Featured Image by Doxepine, Public domain, via Wikimedia Commons.
References
Bahdanau, D., Cho, K., & Bengio, Y. (2014). Neural machine translation by jointly learning to align and translate. arXiv preprint arXiv:1409.0473.
DeepMind. (2020, January 15). AlphaFold: Using AI for scientific discovery. https://deepmind.com/blog/article/AlphaFold-Using-AI-for-scientific-discovery
Cohen, F. E., & Kelly, J. W. (2003). Therapeutic approaches to protein-misfolding diseases. Nature, 426(6968), 905–909. https://doi.org/10.1038/nature02265
He, K., Zhang, X., Ren, S., & Sun, J. (2016). Deep residual learning for image recognition. In Proceedings of the IEEE conference on computer vision and pattern recognition (pp. 770-778).
Jumper, J., Evans, R., Pritzel, A., Green, T., Figurnov, M., Tunyasuvunakool, K., … & Hassabis, D. (2020). High accuracy protein structure prediction using deep learning. Fourteenth Critical Assessment of Techniques for Protein Structure Prediction (Abstract Book), 22, 24.
Levinthal, Cyrus (1969). “How to Fold Graciously”. Mossbauer Spectroscopy in Biological Systems: Proceedings of a meeting held at Allerton House, Monticello, Illinois: 22–24. Archived from the original on 2010-10-07.
Mahy, B. W., Collier, L., Balows, A., & Sussman, M. (1998). Topley and Wilson’s Microbiology and Microbial Infections: Volume 1: Virology (Topley & Wilson’s Microbiology & Microbial Infections) (9th ed.). Hodder Education Publishers.
Moult, J., Pedersen, J. T., Judson, R., & Fidelis, K. (1995). A large-scale experiment to assess protein structure prediction methods. Proteins: Structure, Function, and Genetics, 23(3), ii–iv. https://doi.org/10.1002/prot.340230303
Pellegrini M, Marcotte EM, Thompson MJ, Eisenberg D, Yeates TO. Proc Natl Acad Sci U S A. 1999 Apr 13;96(8):4285-8.
Senior, A. W., Evans, R., Jumper, J., Kirkpatrick, J., Sifre, L., Green, T., Qin, C., Žídek, A., Nelson, A. W. R., Bridgland, A., Penedones, H., Petersen, S., Simonyan, K., Crossan, S., Kohli, P., Jones, D. T., Silver, D., Kavukcuoglu, K., & Hassabis, D. (2020). Improved protein structure prediction using potentials from deep learning. Nature, 577(7792), 706–710. https://doi.org/10.1038/s41586-019-1923-7
Service, R. F. (2020, December 1). ‘The game has changed.’ AI triumphs at solving protein structures. Science | AAAS. https://www.sciencemag.org/news/2020/11/game-has-changed-ai-triumphs-solving-protein-structures
Vaswani, A., Shazeer, N., Parmar, N., Uszkoreit, J., Jones, L., Gomez, A. N., … & Polosukhin, I. (2017). Attention is all you need. arXiv preprint arXiv:1706.03762.
Vig, J., Madani, A., Varshney, L. R., Xiong, C., Socher, R., & Rajani, N. F. (2020). Bertology meets biology: Interpreting attention in protein language models. arXiv preprint arXiv:2006.15222.
Walker, L. C., & LeVine, H. (2000). The cerebral proteopathies: neurodegenerative disorders of protein conformation and assembly. Molecular neurobiology, 21(1-2), 83–95. https://doi.org/10.1385/MN:21:1-2:083
Xie, H., Qin, Z., Li, G. Y., & Juang, B. H. (2021). Deep learning enabled semantic communication systems. IEEE Transactions on Signal Processing, 69, 2663-2675.